WorldPop Projects
Multitemporal Population Mapping
Project leads: Andy Tatem, Andrea Gaughan
Collaborators/funders: Bill and Melinda Gates Foundation, China CDC
Background: The WorldPop project provides datasets at the finer end of the spatial spectrum, at 3 arc seconds (~100 m spatial resolution at the equator), for Africa, Asia and Latin America. These are constructed for the year of the input population data and also for 2010, 2015, and 2020, unadjusted and adjusted using urban and rural growth rates taken from the United Nations World Urbanization Prospects Database to match UN Population Division national total estimates. The WorldPop project also makes available a continually expanding, open-access archive of temporally-comparable, high-resolution datasets of gridded population distribution. These datasets are based on the WorldPop Random Forest-based model and can be used for analyzing population change over time.
Data and methods: Through the assembly of over 100 million records for 87 countries, we have produced age and sex-structured population distribution datasets for the African and Asian continents. On a country-by-country basis, we incorporate the most comprehensive and contemporary data source detailing age and sex structures, including census, micro-census and household surveys.
Outputs: Products include multi-temporal, gridded population datasets unique in terms of granularity and extent, providing fine-scale (~100m) patterns of population distribution on a country-wide basis.
Applications: These datasets provide timely measuring and mapping of residential population patterns decades, generating comparable datasets suitable for analyzing population change across time. To accomplish this task, the model uses a limited set of input data that are time-invariant or temporally-explicit. This approach supports population density and urban definition change analyses in the most robust and accurate manner available at this time. The datasets can be used in support of identifying and modelling populations at risk in epidemiological, climate, and disaster management applications, among others. In contrast to contemporary WorldPop datasets that use a large ancillary set of data in the modeling process, the reduced level of covariates make these population maps decrease the potential for endogeneity in subsequent analyses.
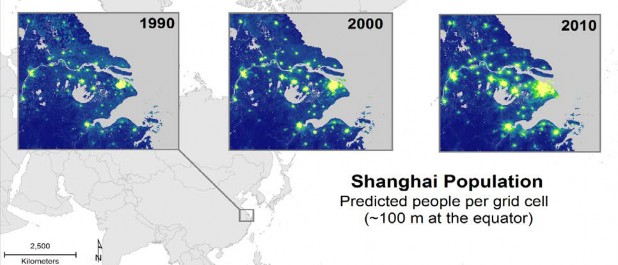