WorldPop Projects
Census Disaggregation for Population Mapping
Project leads: Andy Tatem, Forrest Stevens
Collaborators/funders: Bill and Melinda Gates Foundation, Columbia University, Joint Research Centre of the EC
Contemporary, fine spatial scale, gridded population data is critical across myriad research contexts. We use such data for understanding the ‘now’ at the intersection of hazard risk and mitigation management, health and disease modelling, and economic-, environmental-, and sustainability-related research in relation to population distributions. But to understand how human population shifts through time, and incorporate a more nuanced approach to modelling population change at fine spatial scales, novel ways of treating data and statistically describing associations of measured population counts with associated covariates are needed.
The WorldPop project, now in its twelfth year, is an effort to improve the “denominator,” or the population background against which persistence, transmission and eradication models for various diseases are developed. The high demand for sub-census level estimates of population distribution continues to increase, and with that demand comes a desire for better and more useful data for analyzing change over time. Our research develops leading-edge modelling methods, combining machine learning, cloud-based computing, and the best available census and ancillary data. These data and methods are used to produce 100 meter, gridded population estimates at five year intervals across the tropical and subtropical areas of Latin America, Asia, and Africa.
New efforts supported by funders will include expanding these methods to support annual estimates with global extents. These new ensemble modelling approaches incorporate changing built area environments, multiple years of census data, and multiscalar syntheses that merge data to produce comparable gridded population data across time.
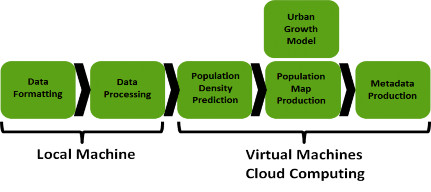
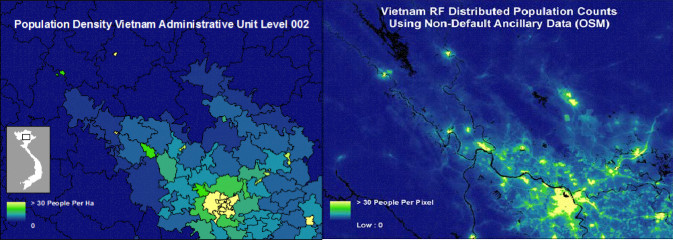
Publications
- High Resolution Population Distribution Maps for Southeast Asia in 2010 and 2015
- Exploring nationally and regionally defined models for large area population mapping
- High-resolution gridded population datasets for Latin America and the Caribbean in 2010, 2015, and 2020
- Random Forest Population Mapping Complexity Reduction Algorithm, Data and Code
- Disaggregating Census Data for Population Mapping Using Random Forests with Remotely-Sensed and Ancillary Data